Tools for Ethical Machine Learning Development
Making use of simple tools to reinforce the importance of ethical development in machine learning can go a long way to ensuring that our solutions meet a standard of fairness and are morally sound. This is particularly important when operating under time constraints or when putting forward a new explorative project. In this post, I'm going to (very briefly) advocate two simple ideas for assisting a more ethically conscious workflow: ethics checklists and model audits.
Ethics checklists
A checklist of ethical questions can act as a concrete reference point, or simply a central motif, from which to spark actionable debate about how to move forward with new or existing machine learning models. Recently, I came across Deon, an open-source Python tool which can be used to add an ethics checklist to a machine learning project. The idea behind this is that we should maintain an
ETHICS.md
document containing an explicit checklist of ethical considerations and precautions taken during the development of our projects. Examples of items in the checklist include user privacy, data biases, and proxy discrimination. This mirrors the README.md
type approach to documentation, which I believe strikes a good balance between simplicity and utility.
The Deon command-line tool makes this actionable by providing a simple interface to generate and customise such an ethics checklist. To use their default checklist (which is a great starting point), simply run the following:
$ pip install deon $ deon -o ETHICS.mdThe default checklist groups 20 items into 5 sections:
- Data Collection
- Data Storage
- Analysis
- Modeling
- Deployment
Model audits
Once an ethics checklist has been set up, one way to assess the fairness of the predictions being made by the model is by an audit. This involves a programmatic assessment of the model predictions as well as the ground truth labels with the aim of uncovering biases or prejudice. From obvious applications, such as medical and autonomous vehicles, through to legal and financial recommendations, the importance of ensuring the fairness of our models is essential. To this end, the Center for Data Science and Public Policy at the University of Chicago has developed a bias and fairness audit tool called Aequitas. Aequitas can be used as a Python library, a command-line tool, or via a web interface to generate a report as well as detailed statistics about biases and the fairness in your model. This assessment is done for a number of bias metrics, defined in the fairness tree.
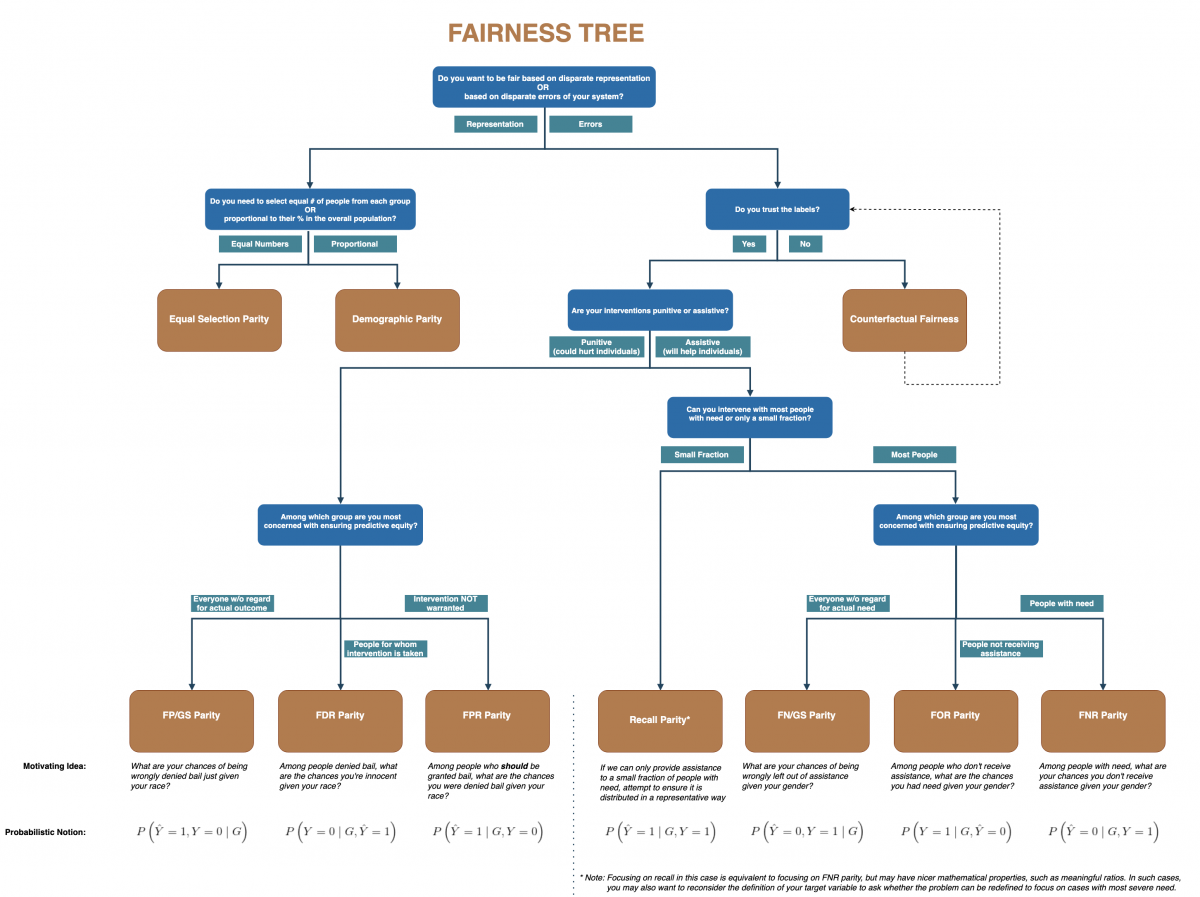
From left to right, the parities depicted in the bottom row of the fairness tree are:
- False Positive
- False Discovery Rate
- False Positive Rate
- Recall
- False Negative
- False Omission Rate
- False Negative Rate
References
Saleiro, Pedro, et al. “Aequitas: A bias and fairness audit toolkit.” arXiv preprint arXiv:1811.05577 (2018).